Recent Advances in Ensembles for Feature Selection: Intelligent Systems for Enhanced Performance

4.5 out of 5
Language | : | English |
File size | : | 9273 KB |
Text-to-Speech | : | Enabled |
Screen Reader | : | Supported |
Enhanced typesetting | : | Enabled |
Print length | : | 219 pages |
Feature selection is a crucial step in machine learning and data mining tasks, as it helps identify the most relevant and informative features from a dataset. This allows for improved model performance, reduced computational costs, and enhanced interpretability.
Ensembles are a powerful technique in machine learning that combine multiple models to achieve better predictive performance. They have been successfully applied to various tasks, including feature selection.
Types of Ensemble Methods for Feature Selection
There are several types of ensemble methods that can be used for feature selection, each with its own strengths and limitations:
- Bagging (Bootstrap Aggregating): Bagging involves creating multiple subsets of the original dataset by randomly sampling with replacement. Each subset is then used to train a base model, and the final prediction is made by combining the predictions of the individual models.
- Boosting: Boosting iteratively trains base models, with each subsequent model focused on correcting the errors of the previous models. The final prediction is made by weighted averaging of the individual model predictions.
- Random Forests: Random forests construct an ensemble of decision trees, where each tree is trained on a different subset of the dataset and a random subset of features. The final prediction is made by majority vote or averaging of the individual tree predictions.
- Hybrid Ensembles: Hybrid ensembles combine different types of ensemble methods to leverage their complementary strengths. For example, a hybrid ensemble could combine bagging and boosting to improve both stability and accuracy.
Advantages of Ensembles for Feature Selection
Ensembles offer several advantages for feature selection:
- Improved Accuracy: By combining the predictions of multiple models, ensembles reduce variance and improve the overall accuracy of feature selection.
- Stability: Ensembles are more stable than individual models, as they are less prone to overfitting and can handle noisy or incomplete data.
- Robustness: Ensembles are robust to outliers and can handle datasets with different feature types and distributions.
- Scalability: Ensembles can be easily parallelized, making them suitable for handling large datasets.
Applications of Ensembles in Intelligent Systems
Ensembles for feature selection have been successfully applied in various intelligent systems, including:
- Medical Diagnosis: Ensembles have been used to identify the most discriminative features for diagnosing diseases, such as cancer and heart disease.
- Image Recognition: Ensembles have helped improve the accuracy of image recognition systems by selecting the most important features for object detection and classification.
- Natural Language Processing: Ensembles have been applied to feature selection for text classification, sentiment analysis, and machine translation.
- Financial Prediction: Ensembles have been used to select the most relevant financial indicators for predicting stock prices and market trends.
Ensembles are a powerful tool for feature selection, offering improved accuracy, stability, robustness, and scalability. By combining the strengths of multiple models, ensembles can enhance the performance of intelligent systems in various applications.
As research in ensemble methods continues, we can expect further advancements in feature selection techniques, leading to even more effective and efficient intelligent systems.
References
- Guyon, I., & Elisseeff, A. (2003). An to variable and feature selection. Journal of Machine Learning Research, 3, 1157-1182.
- Li, J., Cheng, K., Wang, S., Morstatter, F., Trevino, R. P., Tang, J., & Liu, H. (2017). Feature selection: A data perspective. ACM Computing Surveys, 50(6),1-45.
- Fernández-Delgado, M., Cernadas, E., Barro, S., & Amorim, D. (2014). Do we need hundreds of classifiers to solve real world classification problems?. Journal of Machine Learning Research, 15(1),3133-3181.
4.5 out of 5
Language | : | English |
File size | : | 9273 KB |
Text-to-Speech | : | Enabled |
Screen Reader | : | Supported |
Enhanced typesetting | : | Enabled |
Print length | : | 219 pages |
Do you want to contribute by writing guest posts on this blog?
Please contact us and send us a resume of previous articles that you have written.
Book
Chapter
Text
Genre
Library
E-book
Newspaper
Sentence
Bookmark
Glossary
Synopsis
Annotation
Footnote
Scroll
Tome
Bestseller
Classics
Library card
Biography
Autobiography
Memoir
Encyclopedia
Thesaurus
Narrator
Resolution
Catalog
Card Catalog
Archives
Periodicals
Research
Scholarly
Academic
Journals
Rare Books
Literacy
Thesis
Dissertation
Storytelling
Awards
Textbooks
Neil Harrison
Corinne Brown
Larissa Kohler
Paul W Wang
Maya Gottfried
Tim Parks
Caroline Rochford
Martin Kemp
Helen J Knowles
Daisy Hay
George F Luger
Richard M Ketchum
Alistair Maclean
Shawn James
Amy Stewart
Dominik Wexenberger
Dennis Mccarthy
Cat Porter
Steve Kornacki
John Crowley
Light bulbAdvertise smarter! Our strategic ad space ensures maximum exposure. Reserve your spot today!
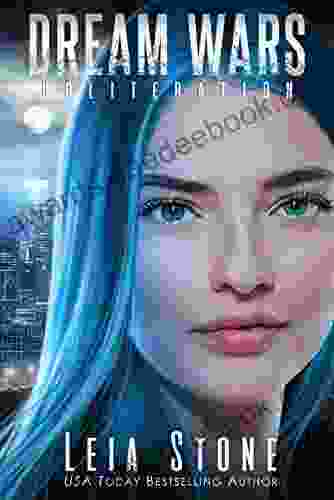

- Shawn ReedFollow ·12.7k
- Deacon BellFollow ·9.6k
- Travis FosterFollow ·6.7k
- Jermaine PowellFollow ·3k
- Carl WalkerFollow ·10.9k
- Dion ReedFollow ·9.8k
- Dashawn HayesFollow ·7.4k
- Greg CoxFollow ·18.1k
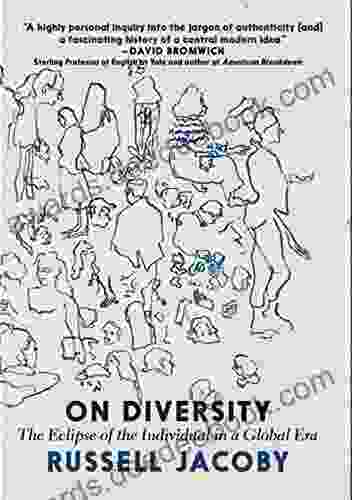

The Waning of the Individual in the Global Era: A...
In the rapidly globalizing world of today,...


First of Verbs: An Early Language
The First of Verbs (FOV) is an early...


The Biography Of The Myth: Routledge Historical...
Who is the Myth?...
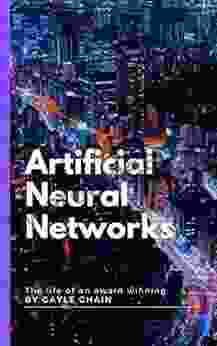

Artificial Neural Networks: Unraveling the Amazing...
Artificial Neural Networks (ANNs),...
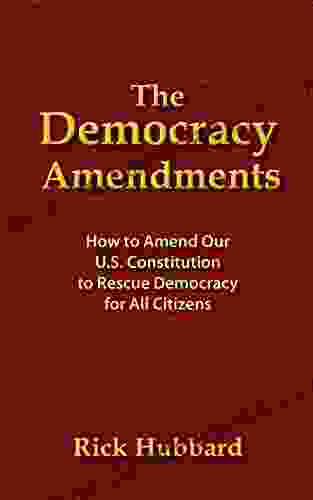

How To Amend Our Constitution To Rescue Democracy For All...
Our democracy is in...
4.5 out of 5
Language | : | English |
File size | : | 9273 KB |
Text-to-Speech | : | Enabled |
Screen Reader | : | Supported |
Enhanced typesetting | : | Enabled |
Print length | : | 219 pages |